National & International Conference
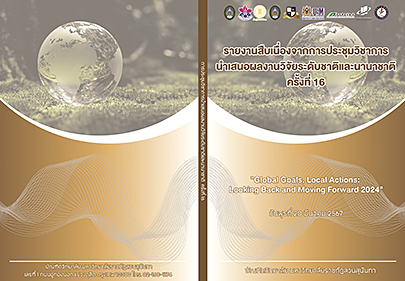
Vol 18, No 1 (2025): 18th International & National Conference
18th International & National Conference
Table of Contents
Cover
cover cover
|
|
Content
content content
|
|
Articles
Le Quoc Hung
|
1
|
Siqi Yao, Suppalak Fakkham, Sarisak Soontornchai
|
7
|
Zhang Xiaohan, Suppalak Fakkham, Sarisak Soontornchai
|
13
|
Anutida Sangsai, Amornrat Muenjitnoy
|
19
|
Jinjutha Senachai, Teerarat Amnartcharoen
|
26
|
Vasuta Auyapitang, Annop Annop T.srivong
|
33
|
Ekkachai Sithamma
|
40
|
Napassawan Kumklong, Srayut Kuanmuang, Surapong Intarapak
|
46
|
Supat Somsuk, Nattachai Aeknarajindawut
|
51
|
Kanlaya Pratapan, Suphakran Sophaporn
|
59
|
THE STUDY OF MARKETING CONTENT FACTORS AND SOCIAL INFLUENCE FROM TIKTOK USERS IN UDON THANI PROVINCE
Annop T.Srivong, Vasuta Auyapitang
|
67
|
Daranee Daungprom, Chalida Srisunthron
|
73
|
Saibua Nonsila, Waleerat Sangchai
|
79
|
Taksa Parakaew, Napassawan Kumklong
|
86
|
Amornrat Muenjitnoy, Phirayu Seanbudda, Nattaya Wongsabut, Suphawee Phakeenuva, Sirion Sutthimethakul
|
93
|
Srayut Kuanmuang, Butsarakham Ampharat
|
100
|
Teerarat Amnartcharoen, Nattawut Kongpangkam, Nattachai Aeknarajindawut
|
107
|
Siqi Yao, Suppalak Fakkham, Sarisak Soontornchai
|
116
|
Thanadol Armartpon, Kanda Teelanont, Teerata Pangkasa
|
122
|
Surapong Intarapak, Butsaraporn Saenchan, Pornchai Yaemban
|
130
|
Waleerat Sangchai, Tawatchai Kualamtan
|
138
|
Chalida Srisunthron, Daranee Daungprom
|
143
|
Suphakarn Sophaporn
|
151
|
Phirayu Seanbudda, Atippatai Noomthursat, Sarawut Utakavapee, Thamakorn Keawsrichun, Nanthasit Anthachai
|
158
|
Pawita Kakhai, Surasak Meebua, Tattanan Khonglamthan
|
165
|
Pornpimon Lorlong, Jutamard Thaweepaiboonwong
|
171
|
Kanda Sarapol, Phirayu Saenbudda
|
180
|
Teerata Pangkasa, Thanadol Armartpon
|
188
|
Michael Jay Labin, Nathanicha Na Nakorn
|
196
|
Yaobang Zhang, Pimporn Thongmuang, Sarisak Soontornchai
|
204
|
Qin Tong, Supalak Fakkham, Sarisak Soontornchai
|
211
|
Nattacha Leepanyaporn, Pawita Kakhai
|
219
|
Tattanan Khonglamthan, Paiboon Chuwatthanakij, Natasorn Auekarn, Nichakorn Khonthong, Surapong Woradit
|
225
|
Wannarat Thongkanta, Tassanee Attharos
|
231
|
Paadsapid Jantachote, Tasanee Satthapong
|
236
|
Sirimanee Banjong, Duangkamol Chongcharoen, Manllika Na Nakorn
|
242
|
Suttinee Chairat, Kamonwan Yoowattana
|
249
|
Shao Linhui, Siriluck Jittrabiab
|
253
|
Thanyalak Thongsuk, Sirimanee Banjong
|
259
|
Jiang Hang, Pimporn Thongmuang, Sarisak Soontornchai
|
265
|
Dipti Kiran, Nathanicha Na Nakorn
|
271
|
Kaung Htike Tun, Papob Puttimanoradeekul, Suthinan Pomsuwan
|
278
|
Supu Xu, Sawitree Santipiriyapon
|
286
|
Yaobang Zhang, Pimporn Thongmuang, Sarisak Soontornchai
|
292
|
Wang Jingjing, Somsak Klaysung
|
297
|
Wang Xiangyu, Somsak Klaysung
|
304
|
Mayu Denta, Atisan Phuwasaktanasiri, Sawitree Santipiriyapon
|
310
|
Jian Wang, Thanawat Imsomboon, Siriluck Jittrabiab
|
318
|
Liugen Wang, Thanawat Imsomboon, Siriluck Jittrabiab
|
325
|
Mariem Sarhali, Atisan Phuwasaktanasiri
|
333
|
Lu Wanhua, Pimporn Thongmuang, Yuttana Sudjaroen
|
340
|
Watcharit Siriudomset, Nuttasorn Ketprapakorn
|
350
|
INFORMATION SYSTEM CONSTRUCTION OF AIDS PREVENTION AND CONTROL IN COLLEGES AND UNIVERSITIES IN SICHU
Jiang Hang, Pimporn Thongmuang, Sarisak Soontornchai
|
357
|
Jian Wang, Thanawat Imsomboon, Siriluck Jittrabiab
|
364
|
Liugen Wang, Thanawat Imsomboon, Siriluck Jittrabiab
|
371
|
Shao Linhui, Siriluck Jittrabiab
|
379
|
Guo Hangru, Thanawat Imsomboon, Siriluck Jittrabiab
|
386
|
Guo Hangru, Thanawat Imsomboon, Siriluck Jittrabiab
|
392
|
Sun Xinghua, Thanasuwit Tabhiranrak
|
398
|
Saba Shohan, Sarinya L. Suttharattanagul
|
407
|
Dang Vi Dat, Nisit Manotungvorapun
|
416
|
Wansu Jaiaree, Chanicha Kidprasert, Kriangkrai Kongseng
|
424
|
Chonnapatana Chirachalermporn, Kriangkrai Kongseng, Chanicha Kidprasert, Jeeranan Sangsrijan
|
430
|
Suphicha Tangsophon, Nuttasorn Ketprapakorn
|
437
|
Zijian Shang, Chutimavadee Thongjeen
|
443
|
Tan Fei, Preecha Wararatchai
|
451
|
Tan Fei, Preecha Wararatchai
|
457
|
Yan Xiaoyu, Papob Puttimanoradeekul, Suthinan Pomsuwan
|
464
|
Jingui Han, Chutimavadee Thongjeen
|
473
|
Zhang YuLong, Preecha Wararatchai
|
481
|
Anouly Laoly, Nisit Manotugnvorapun
|
487
|
Yi Qi, Pordee Sukpun
|
493
|
Chonruitai Kaewrungrueng, Paiboon Chuwatthanakij, Pitsanu Poonpetpun
|
500
|
Paisarn Arunchoknumlap
|
505
|
Jun Wang, Prakaikavin Srijinda
|
513
|
Kamonwan Yoowattana, Chuencheewin Yimfuang, Pattaravis Yoowattana, Sasitorn Chetanont
|
517
|
Pattaravis Yoowattana, Ariya Poorahong, Kungsadan Senachai
|
522
|
Piched Girdwichai, Ekarat Chaichotchaung
|
527
|
Xiaoyu Zhu, Siriluck Jittrabiab, Thanawat Imsomboon, Biyan Wang
|
538
|
Surasak Meebua, Chuencheewin Yimfuang, Tanawat Pisitchinda, Wisa Punyanitya
|
546
|
Tanyaret Boonma, Cholthicha Sudmuk, Suwaree Yordchim, Sarada Jarupan, Kirk Person
|
553
|
Punyawat Panthasarin, Ganratchakan Lertamornsak
|
561
|
Chen Li, Suwaree Yordchim, Suphat Suka Molson, Sarada Jarupan, Kirk Person
|
568
|
Conrad Chan Rafferty, Papob Puttimanoradeekul
|
575
|
Pafun Nilsawas Duhamel, Wijitra Srisorn, Santhan Chayanon
|
582
|
Chutipan Sudduen
|
590
|
Jutamat Sutduean
|
599
|
Mohd Rizaimy Shaharudin, Preecha Wararatchai, Chanthima Maninakha
|
607
|
Wittawin Susutti, Pirun Dilokpatpongsa, Sorawit Tongyib, Pawaton Kaemawichanurat, Wiboonsak Watthayu, Boonkong Dhakonlayodhin, Pornpun Chalermrum, Kochakorn Siriprapai, Prapatsorn Phimnuwong, Attawut Thammachat
|
615
|
Pipat Tanakit
|
624
|
WenYue Deng
|
634
|
Zhihui Tu
|
639
|
Huiyan Luo
|
645
|
Youqian Zhang
|
649
|
Li Huang
|
654
|
Wenrui Li
|
659
|
Yuetong Zhao
|
664
|
Qianyun Chen
|
670
|
Yongjun Lu
|
674
|
Fangfang Zhang
|
682
|
Yanwen Wang
|
688
|
Yuna Zhang
|
692
|
Fangxin Lin
|
697
|
Zhenqiong Xu
|
702
|
Jinyi Liu
|
707
|
Yan Li
|
711
|
Qian Yang
|
716
|
Jinli Zhou
|
721
|
Lin Yuying
|
726
|
Qu Mingming
|
730
|
Feng Caimei
|
736
|
Na Huang
|
741
|
Zhuoxun Li
|
746
|
Huaidan Fan
|
752
|
Zonglei Li
|
757
|
Kang Ye
|
762
|
Yang Yinchao
|
766
|
Zhengwei Li
|
771
|
Shuai Fu
|
776
|
Jiayi Zhu
|
782
|
Ning Wang
|
788
|
Weijian Zheng
|
791
|
Yiyi Zhu
|
796
|
Xinyuan Dong
|
804
|
Pattaphong Satawronsamit, Wijittra Srisorn
|
807
|
Bay Nguyen Van, Suppanat Watcharamathakorn, Arpaporn Phokajang, Thongchai Surinwarangkoon
|
814
|
Euphrasia Pang, Faiza Saleem
|
824
|
Janice Ang Yizing, Sima Ahmadpour, Joshua Teck Khun Loo, Leila Ahmadpour
|
833
|
Patcharaphan Susamawathanakun, Suwaree Yordchim
|
840
|
Phaik-Nie Chin, Gow-Yi Chai, Jiaqi Li
|
845
|
Say Keat Ooi
|
855
|
PREDICTING COLLABORATIVE ARTIFICIAL INTELLIGENCE (AI) ADOPTION AMONG OPERATIONS MANAGERS IN MALAYSIA
Yew Hoo Tan, Ai Ping Teoh
|
862
|
Qin Tong, Supalak Fakkham, Sarisak Soontornchai
|
870
|
Soraya Nimsamoot
|
878
|